Alle Programme verarbeiten Daten in der einen oder anderen Form, und viele müssen in der Lage sein, diese Daten von einem Aufruf zum nächsten zu speichern und abzurufen. Python, SQLite und SQLAlchemy verleihen Ihren Programmen Datenbankfunktionalität, sodass Sie Daten in einer einzigen Datei speichern können, ohne dass ein Datenbankserver erforderlich ist.
Sie können ähnliche Ergebnisse erzielen, indem Sie Flatfiles in einer beliebigen Anzahl von Formaten verwenden, einschließlich CSV, JSON, XML und sogar benutzerdefinierten Formaten. Flatfiles sind häufig für Menschen lesbare Textdateien – obwohl es sich auch um Binärdaten handeln kann – mit einer Struktur, die von einem Computerprogramm analysiert werden kann. Im Folgenden werden Sie die Verwendung von SQL-Datenbanken und Flatfiles für die Datenspeicherung und -bearbeitung untersuchen und lernen, wie Sie entscheiden können, welcher Ansatz der richtige für Ihr Programm ist.
In diesem Tutorial erfahren Sie, wie Sie Folgendes verwenden:
- Flatfiles zur Datenspeicherung
- SQL um den Zugriff auf persistente Daten zu verbessern
- SQLite zur Datenspeicherung
- SQLAlchemy um mit Daten als Python-Objekte zu arbeiten
Sie können den gesamten Code und die Daten, die Sie in diesem Tutorial sehen, abrufen, indem Sie auf den folgenden Link klicken:
Beispielcode herunterladen: Klicken Sie hier, um den Code abzurufen, den Sie verwenden, um in diesem Tutorial mehr über die Datenverwaltung mit SQLite und SQLAlchemy zu erfahren.
Flatfiles zur Datenspeicherung verwenden
Eine flache Datei ist eine Datei, die Daten ohne interne Hierarchie und normalerweise keine Verweise auf externe Dateien enthält. Flatfiles enthalten menschenlesbare Zeichen und sind sehr nützlich zum Erstellen und Lesen von Daten. Da sie keine festen Feldbreiten verwenden müssen, verwenden Flatfiles oft andere Strukturen, um es einem Programm zu ermöglichen, Text zu analysieren.
Dateien mit kommagetrennten Werten (CSV-Dateien) sind beispielsweise reine Textzeilen, in denen das Komma die Datenelemente trennt. Jede Textzeile stellt eine Datenzeile dar, und jeder durch Kommas getrennte Wert ist ein Feld innerhalb dieser Zeile. Das Komma-Trennzeichen gibt die Grenze zwischen Datenwerten an.
Python zeichnet sich durch das Lesen und Speichern in Dateien aus. Da Sie Datendateien mit Python lesen können, können Sie eine Anwendung in einem nützlichen Zustand wiederherstellen, wenn Sie sie zu einem späteren Zeitpunkt erneut ausführen. Durch die Möglichkeit, Daten in einer Datei zu speichern, können Sie Informationen aus dem Programm zwischen Benutzern und Websites austauschen, auf denen die Anwendung ausgeführt wird.
Bevor ein Programm eine Datendatei lesen kann, muss es die Daten verstehen können. Normalerweise bedeutet dies, dass die Datendatei eine Struktur haben muss, die die Anwendung verwenden kann, um den Text in der Datei zu lesen und zu parsen.
Unten ist eine CSV-Datei namens author_book_publisher.csv
, die vom ersten Beispielprogramm in diesem Tutorial verwendet wird:
first_name,last_name,title,publisher
Isaac,Asimov,Foundation,Random House
Pearl,Buck,The Good Earth,Random House
Pearl,Buck,The Good Earth,Simon & Schuster
Tom,Clancy,The Hunt For Red October,Berkley
Tom,Clancy,Patriot Games,Simon & Schuster
Stephen,King,It,Random House
Stephen,King,It,Penguin Random House
Stephen,King,Dead Zone,Random House
Stephen,King,The Shining,Penguin Random House
John,Le Carre,"Tinker, Tailor, Soldier, Spy: A George Smiley Novel",Berkley
Alex,Michaelides,The Silent Patient,Simon & Schuster
Carol,Shaben,Into The Abyss,Simon & Schuster
Die erste Zeile enthält eine durch Kommas getrennte Liste von Feldern, bei denen es sich um die Spaltennamen für die Daten handelt, die in den verbleibenden Zeilen folgen. Die restlichen Zeilen enthalten die Daten, wobei jede Zeile einen einzelnen Datensatz darstellt.
Hinweis: Obwohl die Autoren, Bücher und Verleger alle real sind, sind die Beziehungen zwischen Büchern und Verlegern fiktiv und wurden für die Zwecke dieses Tutorials erstellt.
Als Nächstes werfen Sie einen Blick auf einige der Vor- und Nachteile der Verwendung von Flatfiles wie der obigen CSV-Datei, um mit Ihren Daten zu arbeiten.
Vorteile von Flatfiles
Das Arbeiten mit Daten in Flatfiles ist überschaubar und einfach zu implementieren. Die Daten in einem für Menschen lesbaren Format zu haben, ist nicht nur hilfreich, um die Datendatei mit einem Texteditor zu erstellen, sondern auch, um die Daten zu untersuchen und nach Inkonsistenzen oder Problemen zu suchen.
Viele Anwendungen können Flatfile-Versionen der von der Datei generierten Daten exportieren. Beispielsweise kann Excel eine CSV-Datei in eine und aus einer Tabellenkalkulation importieren oder exportieren. Flatfiles haben auch den Vorteil, dass sie eigenständig und übertragbar sind, wenn Sie die Daten teilen möchten.
Fast jede Programmiersprache verfügt über Tools und Bibliotheken, die die Arbeit mit CSV-Dateien erleichtern. Python hat das eingebaute csv
Modul und das leistungsstarke Pandas-Modul verfügbar, was die Arbeit mit CSV-Dateien zu einer leistungsstarken Lösung macht.
Nachteile von Flatfiles
Die Vorteile der Arbeit mit Flatfiles nehmen mit zunehmender Datenmenge ab. Große Dateien sind immer noch für Menschen lesbar, aber ihre Bearbeitung, um Daten zu erstellen oder nach Problemen zu suchen, wird zu einer schwierigeren Aufgabe. Wenn Ihre Anwendung die Daten in der Datei ändert, besteht eine Lösung darin, die gesamte Datei in den Speicher zu lesen, die Änderungen vorzunehmen und die Daten in eine andere Datei zu schreiben.
Ein weiteres Problem bei der Verwendung von Flatfiles besteht darin, dass Sie alle Beziehungen zwischen Teilen Ihrer Daten und dem Anwendungsprogramm innerhalb der Dateisyntax explizit erstellen und pflegen müssen. Außerdem müssen Sie Code in Ihrer Anwendung generieren, um diese Beziehungen zu verwenden.
Eine letzte Komplikation besteht darin, dass Personen, mit denen Sie Ihre Datendatei teilen möchten, auch die Strukturen und Beziehungen kennen und entsprechend handeln müssen, die Sie in den Daten erstellt haben. Um auf die Informationen zugreifen zu können, müssen diese Benutzer nicht nur die Struktur der Daten verstehen, sondern auch die für den Zugriff erforderlichen Programmiertools.
Flatfile-Beispiel
Das Beispielprogramm examples/example_1/main.py
verwendet die author_book_publisher.csv
Datei, um die darin enthaltenen Daten und Beziehungen zu erhalten. Diese CSV-Datei enthält eine Liste der Autoren, der von ihnen veröffentlichten Bücher und der Herausgeber für jedes der Bücher.
Hinweis: Die in den Beispielen verwendeten Datendateien sind im project/data
verfügbar Verzeichnis. Es gibt auch eine Programmdatei in project/build_data
Verzeichnis, das die Daten generiert. Diese Anwendung ist nützlich, wenn Sie die Daten ändern und zu einem bekannten Zustand zurückkehren möchten.
Um Zugriff auf die in diesem Abschnitt und im gesamten Tutorial verwendeten Datendateien zu erhalten, klicken Sie auf den folgenden Link:
Beispielcode herunterladen: Klicken Sie hier, um den Code abzurufen, den Sie verwenden, um in diesem Tutorial mehr über die Datenverwaltung mit SQLite und SQLAlchemy zu erfahren.
Die oben dargestellte CSV-Datei ist eine ziemlich kleine Datendatei, die nur wenige Autoren, Bücher und Verlage enthält. Sie sollten auch einige Dinge über die Daten beachten:
-
Die Autoren Stephen King und Tom Clancy tauchen mehr als einmal auf, weil mehrere Bücher, die sie veröffentlicht haben, in den Daten vertreten sind.
-
Die Autoren Stephen King und Pearl Buck haben dasselbe Buch von mehr als einem Verlag veröffentlicht.
Diese duplizierten Datenfelder erstellen Beziehungen zwischen anderen Teilen der Daten. Ein Autor kann viele Bücher schreiben und ein Verlag kann mit mehreren Autoren zusammenarbeiten. Autoren und Verleger teilen Beziehungen zu einzelnen Büchern.
Die Beziehungen in author_book_publisher.csv
Datei werden durch Felder dargestellt, die mehrmals in verschiedenen Zeilen der Datendatei erscheinen. Aufgrund dieser Datenredundanz stellen die Daten mehr als eine einzelne zweidimensionale Tabelle dar. Sie werden mehr davon sehen, wenn Sie die Datei verwenden, um eine SQLite-Datenbankdatei zu erstellen.
Das Beispielprogramm examples/example_1/main.py
verwendet die in author_book_publisher.csv
eingebetteten Beziehungen Datei, um einige Daten zu generieren. Es präsentiert zunächst eine Liste der Autoren und die Anzahl der Bücher, die jeder geschrieben hat. Es zeigt dann eine Liste der Verlage und die Anzahl der Autoren, für die jeder Bücher veröffentlicht hat.
Es verwendet auch die treelib
Modul, um eine Baumhierarchie der Autoren, Bücher und Verlage anzuzeigen.
Zuletzt fügt es den Daten ein neues Buch hinzu und zeigt die Baumhierarchie mit dem neuen Buch an Ort und Stelle erneut an. Hier ist das main()
Einstiegsfunktion für dieses Programm:
1def main():
2 """The main entry point of the program"""
3 # Get the resources for the program
4 with resources.path(
5 "project.data", "author_book_publisher.csv"
6 ) as filepath:
7 data = get_data(filepath)
8
9 # Get the number of books printed by each publisher
10 books_by_publisher = get_books_by_publisher(data, ascending=False)
11 for publisher, total_books in books_by_publisher.items():
12 print(f"Publisher: {publisher}, total books: {total_books}")
13 print()
14
15 # Get the number of authors each publisher publishes
16 authors_by_publisher = get_authors_by_publisher(data, ascending=False)
17 for publisher, total_authors in authors_by_publisher.items():
18 print(f"Publisher: {publisher}, total authors: {total_authors}")
19 print()
20
21 # Output hierarchical authors data
22 output_author_hierarchy(data)
23
24 # Add a new book to the data structure
25 data = add_new_book(
26 data,
27 author_name="Stephen King",
28 book_title="The Stand",
29 publisher_name="Random House",
30 )
31
32 # Output the updated hierarchical authors data
33 output_author_hierarchy(data)
Der obige Python-Code führt die folgenden Schritte aus:
- Zeilen 4 bis 7 lesen Sie die
author_book_publisher.csv
Datei in einen Pandas DataFrame. - Zeilen 10 bis 13 die Anzahl der von jedem Verlag veröffentlichten Bücher drucken.
- Zeilen 16 bis 19 Drucken Sie die Anzahl der Autoren, die jedem Verlag zugeordnet sind.
- Zeile 22 gibt die Buchdaten hierarchisch sortiert nach Autoren aus.
- Zeilen 25 bis 30 ein neues Buch zur In-Memory-Struktur hinzufügen.
- Zeile 33 gibt die Buchdaten hierarchisch nach Autoren sortiert aus, inklusive des neu hinzugefügten Buches.
Das Ausführen dieses Programms erzeugt die folgende Ausgabe:
$ python main.py
Publisher: Simon & Schuster, total books: 4
Publisher: Random House, total books: 4
Publisher: Penguin Random House, total books: 2
Publisher: Berkley, total books: 2
Publisher: Simon & Schuster, total authors: 4
Publisher: Random House, total authors: 3
Publisher: Berkley, total authors: 2
Publisher: Penguin Random House, total authors: 1
Authors
├── Alex Michaelides
│ └── The Silent Patient
│ └── Simon & Schuster
├── Carol Shaben
│ └── Into The Abyss
│ └── Simon & Schuster
├── Isaac Asimov
│ └── Foundation
│ └── Random House
├── John Le Carre
│ └── Tinker, Tailor, Soldier, Spy: A George Smiley Novel
│ └── Berkley
├── Pearl Buck
│ └── The Good Earth
│ ├── Random House
│ └── Simon & Schuster
├── Stephen King
│ ├── Dead Zone
│ │ └── Random House
│ ├── It
│ │ ├── Penguin Random House
│ │ └── Random House
│ └── The Shining
│ └── Penguin Random House
└── Tom Clancy
├── Patriot Games
│ └── Simon & Schuster
└── The Hunt For Red October
└── Berkley
Die obige Autorenhierarchie wird in der Ausgabe zweimal dargestellt, mit dem Zusatz von Stephen Kings The Stand , herausgegeben von Random House. Die eigentliche Ausgabe oben wurde bearbeitet und zeigt nur die erste Hierarchieausgabe, um Platz zu sparen.
main()
ruft andere Funktionen auf, um den Großteil der Arbeit zu erledigen. Die erste aufgerufene Funktion ist get_data()
:
def get_data(filepath):
"""Get book data from the csv file"""
return pd.read_csv(filepath)
Diese Funktion übernimmt den Dateipfad zur CSV-Datei und verwendet Pandas, um ihn in einen Pandas-DataFrame einzulesen, den sie dann an den Aufrufer zurückgibt. Der Rückgabewert dieser Funktion wird die Datenstruktur, die an die anderen Funktionen weitergegeben wird, aus denen das Programm besteht.
get_books_by_publisher()
berechnet die Anzahl der von jedem Verlag veröffentlichten Bücher. Die resultierende Pandas-Serie verwendet die GroupBy-Funktion von Pandas, um nach Herausgebern zu gruppieren und dann basierend auf dem ascending
zu sortieren Flagge:
def get_books_by_publisher(data, ascending=True):
"""Return the number of books by each publisher as a pandas series"""
return data.groupby("publisher").size().sort_values(ascending=ascending)
get_authors_by_publisher()
macht im Wesentlichen dasselbe wie die vorherige Funktion, aber für Autoren:
def get_authors_by_publisher(data, ascending=True):
"""Returns the number of authors by each publisher as a pandas series"""
return (
data.assign(name=data.first_name.str.cat(data.last_name, sep=" "))
.groupby("publisher")
.nunique()
.loc[:, "name"]
.sort_values(ascending=ascending)
)
add_new_book()
erstellt ein neues Buch im pandas DataFrame. Der Code prüft, ob der Autor, das Buch oder der Herausgeber bereits vorhanden ist. Wenn nicht, wird ein neues Buch erstellt und an den pandas DataFrame angehängt:
def add_new_book(data, author_name, book_title, publisher_name):
"""Adds a new book to the system"""
# Does the book exist?
first_name, _, last_name = author_name.partition(" ")
if any(
(data.first_name == first_name)
& (data.last_name == last_name)
& (data.title == book_title)
& (data.publisher == publisher_name)
):
return data
# Add the new book
return data.append(
{
"first_name": first_name,
"last_name": last_name,
"title": book_title,
"publisher": publisher_name,
},
ignore_index=True,
)
output_author_hierarchy()
verwendet verschachteltes for
Schleifen, um die Ebenen der Datenstruktur zu durchlaufen. Es verwendet dann die treelib
-Modul, um eine hierarchische Liste der Autoren, der von ihnen veröffentlichten Bücher und der Verlage, die diese Bücher veröffentlicht haben, auszugeben:
def output_author_hierarchy(data):
"""Output the data as a hierarchy list of authors"""
authors = data.assign(
name=data.first_name.str.cat(data.last_name, sep=" ")
)
authors_tree = Tree()
authors_tree.create_node("Authors", "authors")
for author, books in authors.groupby("name"):
authors_tree.create_node(author, author, parent="authors")
for book, publishers in books.groupby("title")["publisher"]:
book_id = f"{author}:{book}"
authors_tree.create_node(book, book_id, parent=author)
for publisher in publishers:
authors_tree.create_node(publisher, parent=book_id)
# Output the hierarchical authors data
authors_tree.show()
Diese Anwendung funktioniert gut und veranschaulicht die Leistung, die Ihnen mit dem Pandas-Modul zur Verfügung steht. Das Modul bietet hervorragende Funktionen zum Lesen einer CSV-Datei und zum Interagieren mit den Daten.
Machen wir weiter und erstellen ein identisch funktionierendes Programm mit Python, einer SQLite-Datenbankversion der Autoren- und Veröffentlichungsdaten und SQLAlchemy, um mit diesen Daten zu interagieren.
Verwenden von SQLite zum Persistieren von Daten
Wie Sie bereits gesehen haben, gibt es redundante Daten in author_book_publisher.csv
Datei. Zum Beispiel alle Informationen zu Pearl Bucks The Good Earth ist doppelt aufgeführt, da das Buch von zwei verschiedenen Verlagen veröffentlicht wurde.
Stellen Sie sich vor, diese Datendatei würde mehr verwandte Daten enthalten, wie die Adresse und Telefonnummer des Autors, Veröffentlichungsdaten und ISBNs für Bücher oder Adressen, Telefonnummern und vielleicht Jahreseinnahmen für Verlage. Diese Daten würden für jedes Stammdatenelement wie Autor, Buch oder Verlag dupliziert.
Es ist möglich, Daten auf diese Weise zu erstellen, aber es wäre äußerst unhandlich. Denken Sie an die Probleme, diese Datendatei aktuell zu halten. Was wäre, wenn Stephen King seinen Namen ändern wollte? Sie müssten mehrere Datensätze mit seinem Namen aktualisieren und sicherstellen, dass keine Tippfehler vorhanden sind.
Schlimmer als die Datenduplizierung wäre die Komplexität des Hinzufügens anderer Beziehungen zu den Daten. Was wäre, wenn Sie sich entscheiden würden, Telefonnummern für die Autoren hinzuzufügen, und sie Telefonnummern für Privat, Arbeit, Handy und vielleicht mehr hätten? Jede neue Beziehung, die Sie für ein beliebiges Stammelement hinzufügen möchten, würde die Anzahl der Datensätze mit der Anzahl der Elemente in dieser neuen Beziehung multiplizieren.
Dieses Problem ist ein Grund dafür, dass Beziehungen in Datenbanksystemen existieren. Ein wichtiges Thema im Datenbank-Engineering ist die Datenbanknormalisierung , oder der Prozess des Aufteilens von Daten, um Redundanz zu reduzieren und die Integrität zu erhöhen. Wenn eine Datenbankstruktur um neue Datentypen erweitert wird, werden durch die vorherige Normalisierung Änderungen an der bestehenden Struktur auf ein Minimum reduziert.
Die SQLite-Datenbank ist in Python verfügbar und wird laut SQLite-Homepage häufiger verwendet als alle anderen Datenbanksysteme zusammen. Es bietet ein voll funktionsfähiges Verwaltungssystem für relationale Datenbanken (RDBMS), das mit einer einzigen Datei arbeitet, um die gesamte Datenbankfunktionalität aufrechtzuerhalten.
Es hat auch den Vorteil, dass kein separater Datenbankserver erforderlich ist, um zu funktionieren. Das Dateiformat der Datenbank ist plattformübergreifend und für jede Programmiersprache zugänglich, die SQLite unterstützt.
All dies sind interessante Informationen, aber wie relevant sind sie für die Verwendung von Flatfiles zur Datenspeicherung? Das erfährst du weiter unten!
Erstellen einer Datenbankstruktur
Der Brute-Force-Ansatz zum Abrufen von author_book_publisher.csv
Daten in eine SQLite-Datenbank würde darin bestehen, eine einzelne Tabelle zu erstellen, die der Struktur der CSV-Datei entspricht. Dadurch würde ein Großteil der Leistungsfähigkeit von SQLite ignoriert.
Relationale Datenbanken bieten eine Möglichkeit, strukturierte Daten in Tabellen zu speichern und Beziehungen zwischen diesen Tabellen herzustellen. Sie verwenden normalerweise die strukturierte Abfragesprache (SQL) als primäre Methode zur Interaktion mit den Daten. Dies ist eine zu starke Vereinfachung dessen, was RDBMSs bieten, aber für die Zwecke dieses Tutorials ist es ausreichend.
Eine SQLite-Datenbank bietet Unterstützung für die Interaktion mit der Datentabelle mithilfe von SQL. Eine SQLite-Datenbankdatei enthält nicht nur die Daten, sondern verfügt auch über eine standardisierte Methode zur Interaktion mit den Daten. Diese Unterstützung ist in die Datei eingebettet, was bedeutet, dass jede Programmiersprache, die eine SQLite-Datei verwenden kann, auch SQL verwenden kann, um damit zu arbeiten.
Interaktion mit einer Datenbank mit SQL
SQL ist eine deklarative Sprache Wird verwendet, um die in einer Datenbank enthaltenen Daten zu erstellen, zu verwalten und abzufragen. Eine deklarative Sprache beschreibt was zu bewerkstelligen ist und nicht wie es sollte vollbracht werden. Sie werden später Beispiele für SQL-Anweisungen sehen, wenn Sie mit dem Erstellen von Datenbanktabellen beginnen.
Strukturieren einer Datenbank mit SQL
Um die Vorteile von SQL zu nutzen, müssen Sie eine gewisse Datenbanknormalisierung auf die Daten in author_book_publisher.csv
anwenden Datei. Dazu trennen Sie die Autoren, Bücher und Verlage in separate Datenbanktabellen.
Konzeptionell werden Daten in der Datenbank in zweidimensionalen Tabellenstrukturen gespeichert. Jede Tabelle besteht aus Reihen von Datensätzen , und jeder Datensatz besteht aus Spalten oder Feldern , enthält Daten.
Die in den Feldern enthaltenen Daten sind vordefinierte Typen, einschließlich Text, Ganzzahlen, Gleitkommazahlen und mehr. CSV-Dateien sind anders, da alle Felder Textfelder sind und von einem Programm geparst werden müssen, damit ihnen ein Datentyp zugewiesen wird.
Jeder Datensatz in der Tabelle hat einen Primärschlüssel definiert, um einem Datensatz eine eindeutige Kennung zu geben. Der Primärschlüssel ähnelt dem Schlüssel in einem Python-Wörterbuch. Die Datenbank-Engine selbst generiert oft den Primärschlüssel als inkrementierenden ganzzahligen Wert für jeden Datensatz, der in die Datenbanktabelle eingefügt wird.
Obwohl der Primärschlüssel häufig automatisch von der Datenbank-Engine generiert wird, muss dies nicht der Fall sein. Wenn die in einem Feld gespeicherten Daten für alle anderen Daten in der Tabelle in diesem Feld eindeutig sind, kann es sich um den Primärschlüssel handeln. Beispielsweise könnte eine Tabelle mit Daten zu Büchern die ISBN des Buchs als Primärschlüssel verwenden.
Tabellen mit SQL erstellen
So können Sie mithilfe von SQL-Anweisungen die drei Tabellen erstellen, die die Autoren, Bücher und Verlage in der CSV-Datei darstellen:
CREATE TABLE author (
author_id INTEGER NOT NULL PRIMARY KEY,
first_name VARCHAR,
last_name VARCHAR
);
CREATE TABLE book (
book_id INTEGER NOT NULL PRIMARY KEY,
author_id INTEGER REFERENCES author,
title VARCHAR
);
CREATE TABLE publisher (
publisher_id INTEGER NOT NULL PRIMARY KEY,
name VARCHAR
);
Beachten Sie, dass keine Dateioperationen, keine Variablen erstellt und keine Strukturen, um sie zu halten, vorhanden sind. Die Anweisungen beschreiben nur das gewünschte Ergebnis:die Erstellung einer Tabelle mit bestimmten Attributen. Die Datenbank-Engine bestimmt, wie dies zu tun ist.
Sobald Sie diese Tabelle erstellt und mit Autorendaten aus author_book_publisher.csv
gefüllt haben Datei können Sie mit SQL-Anweisungen darauf zugreifen. Die folgende Anweisung (auch als Abfrage bezeichnet ) verwendet das Platzhalterzeichen (*
), um alle Daten im author
zu erhalten Tabelle und gib sie aus:
SELECT * FROM author;
Sie können das sqlite3
verwenden Befehlszeilentool zur Interaktion mit author_book_publisher.db
Datenbankdatei im project/data
Verzeichnis:
$ sqlite3 author_book_publisher.db
Sobald das SQLite-Befehlszeilentool mit geöffneter Datenbank ausgeführt wird, können Sie SQL-Befehle eingeben. Hier ist der obige SQL-Befehl und seine Ausgabe, gefolgt von .q
Befehl zum Beenden des Programms:
sqlite> SELECT * FROM author;
1|Isaac|Asimov
2|Pearl|Buck
3|Tom|Clancy
4|Stephen|King
5|John|Le Carre
6|Alex|Michaelides
7|Carol|Shaben
sqlite> .q
Beachten Sie, dass jeder Autor nur einmal in der Tabelle vorhanden ist. Im Gegensatz zur CSV-Datei, die für einige Autoren mehrere Einträge hatte, ist hier nur ein eindeutiger Datensatz pro Autor erforderlich.
Pflege einer Datenbank mit SQL
SQL bietet Möglichkeiten, mit vorhandenen Datenbanken und Tabellen zu arbeiten, indem neue Daten eingefügt und vorhandene Daten aktualisiert oder gelöscht werden. Hier ist eine beispielhafte SQL-Anweisung zum Einfügen eines neuen Autors in den author
Tabelle:
INSERT INTO author
(first_name, last_name)
VALUES ('Paul', 'Mendez');
Diese SQL-Anweisung fügt die Werte ‚Paul
ein ‘ und ‚Mendez
‘ in die jeweiligen Spalten first_name
und last_name
des author
Tisch.
Beachten Sie, dass die author_id
Spalte ist nicht angegeben. Da diese Spalte der Primärschlüssel ist, generiert die Datenbank-Engine den Wert und fügt ihn als Teil der Anweisungsausführung ein.
Das Aktualisieren von Datensätzen in einer Datenbanktabelle ist ein unkomplizierter Vorgang. Angenommen, Stephen King wollte unter seinem Pseudonym Richard Bachman bekannt sein. Hier ist eine SQL-Anweisung zum Aktualisieren des Datenbankeintrags:
UPDATE author
SET first_name = 'Richard', last_name = 'Bachman'
WHERE first_name = 'Stephen' AND last_name = 'King';
Die SQL-Anweisung sucht den einzelnen Datensatz für 'Stephen King'
mit der bedingten Anweisung WHERE first_name = 'Stephen' AND last_name = 'King'
und aktualisiert dann den first_name
und last_name
Felder mit den neuen Werten. SQL verwendet das Gleichheitszeichen (=
) sowohl als Vergleichsoperator als auch als Zuweisungsoperator.
Sie können auch Datensätze aus einer Datenbank löschen. Hier ist eine Beispiel-SQL-Anweisung zum Löschen eines Datensatzes aus dem author
Tabelle:
DELETE FROM author
WHERE first_name = 'Paul'
AND last_name = 'Mendez';
Diese SQL-Anweisung löscht eine einzelne Zeile aus author
Tabelle, in der der first_name
ist gleich 'Paul'
und der last_name
ist gleich 'Mendez'
.
Vorsicht beim Löschen von Datensätzen! Die Bedingungen, die Sie festlegen, müssen so spezifisch wie möglich sein. Eine zu breite Bedingung kann dazu führen, dass mehr Datensätze gelöscht werden als beabsichtigt. Zum Beispiel, wenn die Bedingung nur auf der Zeile first_name = 'Paul'
basiert , dann würden alle Autoren mit dem Vornamen Paul aus der Datenbank gelöscht.
Hinweis: Um das versehentliche Löschen von Datensätzen zu vermeiden, erlauben viele Anwendungen überhaupt keine Löschungen. Stattdessen hat der Datensatz eine weitere Spalte, um anzugeben, ob er verwendet wird oder nicht. Diese Spalte könnte den Namen active
haben und enthalten einen Wert, der entweder True oder False ergibt und angibt, ob der Datensatz beim Abfragen der Datenbank eingeschlossen werden soll.
Die folgende SQL-Abfrage würde beispielsweise alle Spalten für alle aktiven Datensätze in some_table
abrufen :
SELECT
*
FROM some_table
WHERE active = 1;
SQLite hat keinen booleschen Datentyp, also active
Spalte wird durch eine Ganzzahl mit dem Wert 0
dargestellt oder 1
um den Status der Aufzeichnung anzuzeigen. Andere Datenbanksysteme können native boolesche Datentypen haben oder auch nicht.
Es ist durchaus möglich, Datenbankanwendungen in Python mit SQL-Anweisungen direkt im Code zu erstellen. Dadurch werden Daten als Liste von Listen oder Wörterbüchern an die Anwendung zurückgegeben.
Die Verwendung von Raw-SQL ist eine durchaus akzeptable Methode, um mit den Daten zu arbeiten, die von Abfragen an die Datenbank zurückgegeben werden. Anstatt dies zu tun, werden Sie jedoch direkt zur Verwendung von SQLAlchemy übergehen, um mit Datenbanken zu arbeiten.
Beziehungen aufbauen
Eine weitere Funktion von Datenbanksystemen, die Sie möglicherweise noch leistungsfähiger und nützlicher finden als Datenpersistenz und -abruf, sind Beziehungen . Datenbanken, die Beziehungen unterstützen, ermöglichen es Ihnen, Daten in mehrere Tabellen aufzuteilen und Verbindungen zwischen ihnen herzustellen.
Die Daten in der author_book_publisher.csv
Datei repräsentiert die Daten und Beziehungen durch Duplizieren von Daten. Eine Datenbank handhabt dies, indem sie die Daten in drei Tabellen aufteilt – author
, book
, und publisher
– und Aufbau von Beziehungen zwischen ihnen.
Nachdem Sie alle gewünschten Daten an einer Stelle in der CSV-Datei gesammelt haben, warum sollten Sie sie dann in mehrere Tabellen aufteilen? Wäre es nicht mehr Arbeit, sie zu erstellen und wieder zusammenzusetzen? Das stimmt bis zu einem gewissen Grad, aber die Vorteile, die Daten aufzubrechen und mit SQL wieder zusammenzusetzen, könnten Sie überzeugen!
Eins-zu-Viele-Beziehungen
Ein Eins-zu-Vielen Beziehung ist wie die eines Kunden, der Artikel online bestellt. Ein Kunde kann viele Bestellungen haben, aber jede Bestellung gehört zu einem Kunden. Die author_book_publisher.db
Datenbank hat eine Eins-zu-Viele-Beziehung in Form von Autoren und Büchern. Jeder Autor kann viele Bücher schreiben, aber jedes Buch wird von einem Autor geschrieben.
Wie Sie oben bei der Tabellenerstellung gesehen haben, besteht die Implementierung dieser separaten Entitäten darin, jede in eine Datenbanktabelle zu platzieren, eine für Autoren und eine für Bücher. Aber wie wird die 1:n-Beziehung zwischen diesen beiden Tabellen implementiert?
Denken Sie daran, dass jede Tabelle in einer Datenbank ein Feld hat, das als Primärschlüssel für diese Tabelle bestimmt ist. Jede obige Tabelle hat ein Primärschlüsselfeld, das nach diesem Muster benannt ist:<table name>_id
.
Das book
Die oben gezeigte Tabelle enthält ein Feld, author_id
, die auf den author
verweist Tisch. Die author_id
-Feld stellt eine 1:n-Beziehung zwischen Autoren und Büchern her, die wie folgt aussieht:

Das obige Diagramm ist ein einfaches Entity-Relationship-Diagramm (ERD), das mit der JetBrains DataGrip-Anwendung erstellt wurde und die Tabellen author
zeigt und book
als Boxen mit ihren jeweiligen Primärschlüssel- und Datenfeldern. Zwei grafische Elemente fügen Informationen über die Beziehung hinzu:
-
Die kleinen gelben und blauen Schlüsselsymbole Geben Sie den Primär- bzw. den Fremdschlüssel für die Tabelle an.
-
Der Pfeil, der
book
verbindet anauthor
gibt die Beziehung zwischen den Tabellen basierend auf derauthor_id
an Fremdschlüssel imbook
Tabelle.
Wenn Sie ein neues Buch zum book
hinzufügen Tabelle enthalten die Daten eine author_id
Wert für einen bestehenden Autor im author
Tisch. Auf diese Weise haben alle von einem Autor geschriebenen Bücher eine Nachschlagebeziehung zu diesem eindeutigen Autor.
Nun, da Sie separate Tabellen für Autoren und Bücher haben, wie nutzen Sie die Beziehung zwischen ihnen? SQL unterstützt einen sogenannten JOIN
-Operation, mit der Sie der Datenbank mitteilen können, wie zwei oder mehr Tabellen verbunden werden sollen.
Die folgende SQL-Abfrage schließt sich dem author
an und book
Tabelle zusammen mit der SQLite-Befehlszeilenanwendung:
sqlite> SELECT
...> a.first_name || ' ' || a.last_name AS author_name,
...> b.title AS book_title
...> FROM author a
...> JOIN book b ON b.author_id = a.author_id
...> ORDER BY a.last_name ASC;
Isaac Asimov|Foundation
Pearl Buck|The Good Earth
Tom Clancy|The Hunt For Red October
Tom Clancy|Patriot Games
Stephen King|It
Stephen King|Dead Zone
Stephen King|The Shining
John Le Carre|Tinker, Tailor, Soldier, Spy: A George Smiley Novel
Alex Michaelides|The Silent Patient
Carol Shaben|Into The Abyss
Die obige SQL-Abfrage sammelt Informationen sowohl aus der Autoren- als auch aus der Buchtabelle, indem die Tabellen mithilfe der zwischen den beiden hergestellten Beziehung verknüpft werden. Die SQL-String-Verkettung weist dem Alias author_name
den vollständigen Namen des Autors zu . Die von der Abfrage gesammelten Daten werden in aufsteigender Reihenfolge nach last_name
sortiert Feld.
Bei der SQL-Anweisung sind einige Dinge zu beachten. Zunächst werden Autoren mit ihren vollständigen Namen in einer einzigen Spalte präsentiert und nach ihren Nachnamen sortiert. Außerdem erscheinen Autoren aufgrund der 1:n-Beziehung mehrfach in der Ausgabe. Der Name eines Autors wird für jedes Buch, das er in der Datenbank geschrieben hat, dupliziert.
Indem Sie separate Tabellen für Autoren und Bücher erstellen und die Beziehung zwischen ihnen herstellen, haben Sie die Redundanz in den Daten reduziert. Jetzt müssen Sie die Daten eines Autors nur noch an einer Stelle bearbeiten, und diese Änderung erscheint in jeder SQL-Abfrage, die auf die Daten zugreift.
Many-to-Many-Beziehungen
Many-to-Many Beziehungen bestehen in author_book_publisher.db
Datenbank zwischen Autoren und Verlegern sowie zwischen Büchern und Verlegern. Ein Autor kann mit vielen Verlagen zusammenarbeiten, und ein Verlag kann mit vielen Autoren zusammenarbeiten. Ebenso kann ein Buch von vielen Verlagen veröffentlicht werden und ein Verlag kann viele Bücher veröffentlichen.
Handling this situation in the database is more involved than a one-to-many relationship because the relationship goes both ways. Many-to-many relationships are created by an association table acting as a bridge between the two related tables.
The association table contains at least two foreign key fields, which are the primary keys of each of the two associated tables. This SQL statement creates the association table relating the author
and publisher
tables:
CREATE TABLE author_publisher (
author_id INTEGER REFERENCES author,
publisher_id INTEGER REFERENCES publisher
);
The SQL statements create a new author_publisher
table referencing the primary keys of the existing author
and publisher
tables. The author_publisher
table is an association table establishing relationships between an author and a publisher.
Because the relationship is between two primary keys, there’s no need to create a primary key for the association table itself. The combination of the two related keys creates a unique identifier for a row of data.
As before, you use the JOIN
keyword to connect two tables together. Connecting the author
table to the publisher
table is a two-step process:
JOIN
theauthor
table with theauthor_publisher
table.JOIN
theauthor_publisher
table with thepublisher
table.
The author_publisher
association table provides the bridge through which the JOIN
connects the two tables. Here’s an example SQL query returning a list of authors and the publishers publishing their books:
1sqlite> SELECT
2 ...> a.first_name || ' ' || a.last_name AS author_name,
3 ...> p.name AS publisher_name
4 ...> FROM author a
5 ...> JOIN author_publisher ap ON ap.author_id = a.author_id
6 ...> JOIN publisher p ON p.publisher_id = ap.publisher_id
7 ...> ORDER BY a.last_name ASC;
8Isaac Asimov|Random House
9Pearl Buck|Random House
10Pearl Buck|Simon & Schuster
11Tom Clancy|Berkley
12Tom Clancy|Simon & Schuster
13Stephen King|Random House
14Stephen King|Penguin Random House
15John Le Carre|Berkley
16Alex Michaelides|Simon & Schuster
17Carol Shaben|Simon & Schuster
The statements above perform the following actions:
-
Line 1 starts a
SELECT
statement to get data from the database. -
Line 2 selects the first and last name from the
author
table using thea
alias for theauthor
table and concatenates them together with a space character. -
Line 3 selects the publisher’s name aliased to
publisher_name
. -
Line 4 uses the
author
table as the first source from which to retrieve data and assigns it to the aliasa
. -
Line 5 is the first step of the process outlined above for connecting the
author
table to thepublisher
table. It uses the aliasap
for theauthor_publisher
association table and performs aJOIN
operation to connect theap.author_id
foreign key reference to thea.author_id
primary key in theauthor
table. -
Line 6 is the second step in the two-step process mentioned above. It uses the alias
p
for thepublisher
table and performs aJOIN
operation to relate theap.publisher_id
foreign key reference to thep.publisher_id
primary key in thepublisher
table. -
Line 7 sorts the data by the author’s last name in ascending alphabetical order and ends the SQL query.
-
Lines 8 to 17 are the output of the SQL query.
Note that the data in the source author
and publisher
tables are normalized, with no data duplication. Yet the returned results have duplicated data where necessary to answer the SQL query.
The SQL query above demonstrates how to make use of a relationship using the SQL JOIN
keyword, but the resulting data is a partial re-creation of the author_book_publisher.csv
CSV data. What’s the win for having done the work of creating a database to separate the data?
Here’s another SQL query to show a little bit of the power of SQL and the database engine:
1sqlite> SELECT
2 ...> a.first_name || ' ' || a.last_name AS author_name,
3 ...> COUNT(b.title) AS total_books
4 ...> FROM author a
5 ...> JOIN book b ON b.author_id = a.author_id
6 ...> GROUP BY author_name
7 ...> ORDER BY total_books DESC, a.last_name ASC;
8Stephen King|3
9Tom Clancy|2
10Isaac Asimov|1
11Pearl Buck|1
12John Le Carre|1
13Alex Michaelides|1
14Carol Shaben|1
The SQL query above returns the list of authors and the number of books they’ve written. The list is sorted first by the number of books in descending order, then by the author’s name in alphabetical order:
-
Line 1 begins the SQL query with the
SELECT
keyword. -
Line 2 selects the author’s first and last names, separated by a space character, and creates the alias
author_name
. -
Line 3 counts the number of books written by each author, which will be used later by the
ORDER BY
clause to sort the list. -
Line 4 selects the
author
table to get data from and creates thea
alias. -
Line 5 connects to the related
book
table through aJOIN
to theauthor_id
and creates theb
alias for thebook
table. -
Line 6 generates the aggregated author and total number of books data by using the
GROUP BY
keyword.GROUP BY
is what groups eachauthor_name
and controls what books are tallied byCOUNT()
for that author. -
Line 7 sorts the output first by number of books in descending order, then by the author’s last name in ascending alphabetical order.
-
Lines 8 to 14 are the output of the SQL query.
In the above example, you take advantage of SQL to perform aggregation calculations and sort the results into a useful order. Having the database perform calculations based on its built-in data organization ability is usually faster than performing the same kinds of calculations on raw data sets in Python. SQL offers the advantages of using set theory embedded in RDBMS databases.
Entity Relationship Diagrams
An entity-relationship diagram (ERD) is a visual depiction of an entity-relationship model for a database or part of a database. The author_book_publisher.db
SQLite database is small enough that the entire database can be visualized by the diagram shown below:
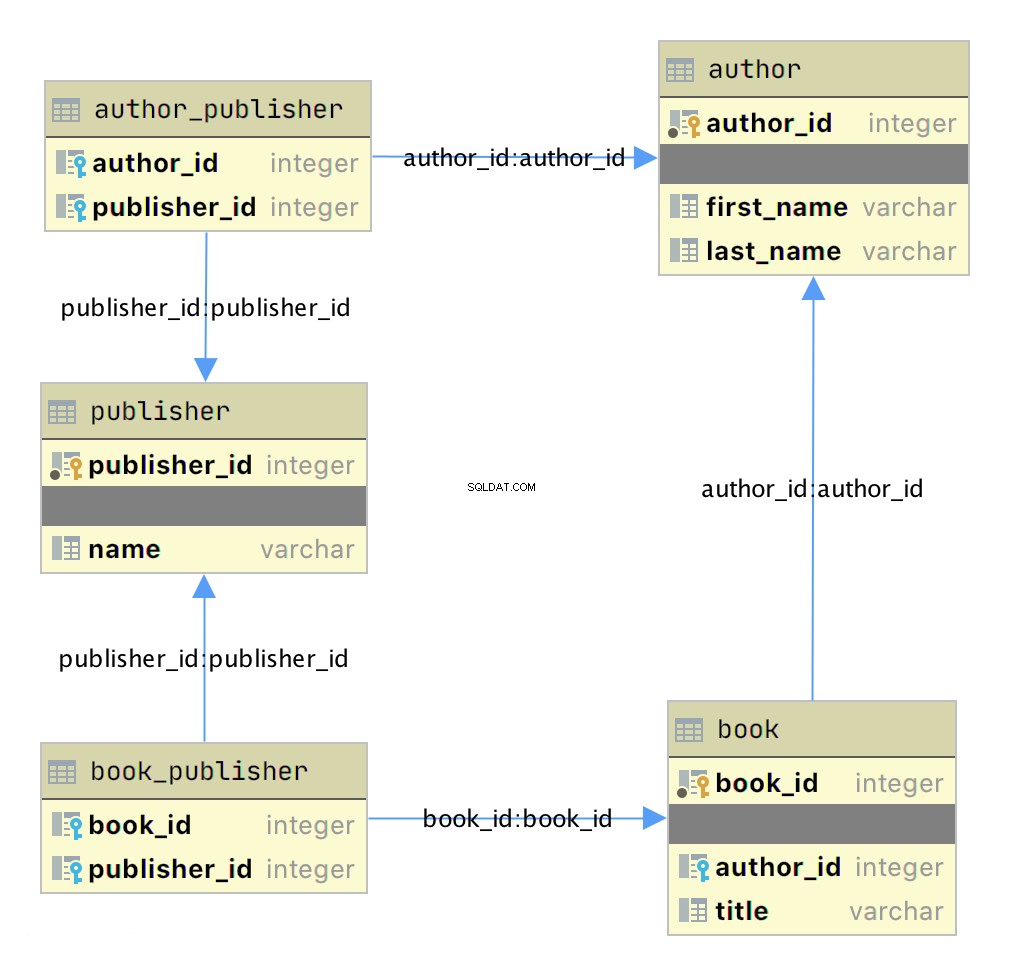
This diagram presents the table structures in the database and the relationships between them. Each box represents a table and contains the fields defined in the table, with the primary key indicated first if it exists.
The arrows show the relationships between the tables connecting a foreign key field in one table to a field, often the primary key, in another table. The table book_publisher
has two arrows, one connecting it to the book
table and another connecting it to the publisher
table. The arrow indicates the many-to-many relationship between the book
and publisher
tables. The author_publisher
table provides the same relationship between author
and publisher
.
Working With SQLAlchemy and Python Objects
SQLAlchemy is a powerful database access tool kit for Python, with its object-relational mapper (ORM) being one of its most famous components, and the one discussed and used here.
When you’re working in an object-oriented language like Python, it’s often useful to think in terms of objects. It’s possible to map the results returned by SQL queries to objects, but doing so works against the grain of how the database works. Sticking with the scalar results provided by SQL works against the grain of how Python developers work. This problem is known as object-relational impedance mismatch.
The ORM provided by SQLAlchemy sits between the SQLite database and your Python program and transforms the data flow between the database engine and Python objects. SQLAlchemy allows you to think in terms of objects and still retain the powerful features of a database engine.
The Model
One of the fundamental elements to enable connecting SQLAlchemy to a database is creating a model . The model is a Python class defining the data mapping between the Python objects returned as a result of a database query and the underlying database tables.
The entity-relationship diagram displayed earlier shows boxes connected with arrows. The boxes are the tables built with the SQL commands and are what the Python classes will model. The arrows are the relationships between the tables.
The models are Python classes inheriting from an SQLAlchemy Base
Klasse. The Base
class provides the interface operations between instances of the model and the database table.
Below is the models.py
file that creates the models to represent the author_book_publisher.db
database:
1from sqlalchemy import Column, Integer, String, ForeignKey, Table
2from sqlalchemy.orm import relationship, backref
3from sqlalchemy.ext.declarative import declarative_base
4
5Base = declarative_base()
6
7author_publisher = Table(
8 "author_publisher",
9 Base.metadata,
10 Column("author_id", Integer, ForeignKey("author.author_id")),
11 Column("publisher_id", Integer, ForeignKey("publisher.publisher_id")),
12)
13
14book_publisher = Table(
15 "book_publisher",
16 Base.metadata,
17 Column("book_id", Integer, ForeignKey("book.book_id")),
18 Column("publisher_id", Integer, ForeignKey("publisher.publisher_id")),
19)
20
21class Author(Base):
22 __tablename__ = "author"
23 author_id = Column(Integer, primary_key=True)
24 first_name = Column(String)
25 last_name = Column(String)
26 books = relationship("Book", backref=backref("author"))
27 publishers = relationship(
28 "Publisher", secondary=author_publisher, back_populates="authors"
29 )
30
31class Book(Base):
32 __tablename__ = "book"
33 book_id = Column(Integer, primary_key=True)
34 author_id = Column(Integer, ForeignKey("author.author_id"))
35 title = Column(String)
36 publishers = relationship(
37 "Publisher", secondary=book_publisher, back_populates="books"
38 )
39
40class Publisher(Base):
41 __tablename__ = "publisher"
42 publisher_id = Column(Integer, primary_key=True)
43 name = Column(String)
44 authors = relationship(
45 "Author", secondary=author_publisher, back_populates="publishers"
46 )
47 books = relationship(
48 "Book", secondary=book_publisher, back_populates="publishers"
49 )
Here’s what’s going on in this module:
-
Line 1 imports the
Column
,Integer
,String
,ForeignKey
, andTable
classes from SQLAlchemy, which are used to help define the model attributes. -
Line 2 imports the
relationship()
andbackref
objects, which are used to create the relationships between objects. -
Line 3 imports the
declarative_base
object, which connects the database engine to the SQLAlchemy functionality of the models. -
Line 5 creates the
Base
class, which is what all models inherit from and how they get SQLAlchemy ORM functionality. -
Lines 7 to 12 create the
author_publisher
association table model. -
Lines 14 to 19 create the
book_publisher
association table model. -
Lines 21 to 29 define the
Author
class model to theauthor
database table. -
Lines 31 to 38 define the
Book
class model to thebook
database table. -
Lines 40 to 49 define the
Publisher
class model to thepublisher
database table.
The description above shows the mapping of the five tables in the author_book_publisher.db
Datenbank. But it glosses over some SQLAlchemy ORM features, including Table
, ForeignKey
, relationship()
, and backref
. Let’s get into those now.
Table
Creates Associations
author_publisher
and book_publisher
are both instances of the Table
class that create the many-to-many association tables used between the author
and publisher
tables and the book
and publisher
tables, respectively.
The SQLAlchemy Table
class creates a unique instance of an ORM mapped table within the database. The first parameter is the table name as defined in the database, and the second is Base.metadata
, which provides the connection between the SQLAlchemy functionality and the database engine.
The rest of the parameters are instances of the Column
class defining the table fields by name, their type, and in the example above, an instance of a ForeignKey
.
ForeignKey
Creates a Connection
The SQLAlchemy ForeignKey
class defines a dependency between two Column
fields in different tables. A ForeignKey
is how you make SQLAlchemy aware of the relationships between tables. For example, this line from the author_publisher
instance creation establishes a foreign key relationship:
Column("author_id", Integer, ForeignKey("author.author_id"))
The statement above tells SQLAlchemy that there’s a column in the author_publisher
table named author_id
. The type of that column is Integer
, and author_id
is a foreign key related to the primary key in the author
table.
Having both author_id
and publisher_id
defined in the author_publisher
Table
instance creates the connection from the author
table to the publisher
table and vice versa, establishing a many-to-many relationship.
relationship()
Establishes a Collection
Having a ForeignKey
defines the existence of the relationship between tables but not the collection of books an author can have. Take a look at this line in the Author
class definition:
books = relationship("Book", backref=backref("author"))
The code above defines a parent-child collection. The books
attribute being plural (which is not a requirement, just a convention) is an indication that it’s a collection.
The first parameter to relationship()
, the class name Book
(which is not the table name book
), is the class to which the books
attribute is related. The relationship
informs SQLAlchemy that there’s a relationship between the Author
and Book
classes. SQLAlchemy will find the relationship in the Book
class definition:
author_id = Column(Integer, ForeignKey("author.author_id"))
SQLAlchemy recognizes that this is the ForeignKey
connection point between the two classes. You’ll get to the backref
parameter in relationship()
in a moment.
The other relationship in Author
is to the Publisher
Klasse. This is created with the following statement in the Author
class definition:
publishers = relationship(
"Publisher", secondary=author_publisher, back_populates="authors"
)
Like books
, the attribute publishers
indicates a collection of publishers associated with an author. The first parameter, "Publisher"
, informs SQLAlchemy what the related class is. The second and third parameters are secondary=author_publisher
and back_populates="authors"
:
-
secondary
tells SQLAlchemy that the relationship to thePublisher
class is through a secondary table, which is theauthor_publisher
association table created earlier inmodels.py
. Thesecondary
parameter makes SQLAlchemy find thepublisher_id
ForeignKey
defined in theauthor_publisher
association table. -
back_populates
is a convenience configuration telling SQLAlchemy that there’s a complementary collection in thePublisher
class calledauthors
.
backref
Mirrors Attributes
The backref
parameter of the books
collection relationship()
creates an author
attribute for each Book
instance. This attribute refers to the parent Author
that the Book
instance is related to.
For example, if you executed the following Python code, then a Book
instance would be returned from the SQLAlchemy query. The Book
instance has attributes that can be used to print out information about the book:
book = session.query(Book).filter_by(Book.title == "The Stand").one_or_none()
print(f"Authors name: {book.author.first_name} {book.author.last_name}")
The existence of the author
attribute in the Book
above is because of the backref
definition. A backref
can be very handy to have when you need to refer to the parent and all you have is a child instance.
Queries Answer Questions
You can make a basic query like SELECT * FROM author;
in SQLAlchemy like this:
results = session.query(Author).all()
The session
is an SQLAlchemy object used to communicate with SQLite in the Python example programs. Here, you tell the session you want to execute a query against the Author
model and return all records.
At this point, the advantages of using SQLAlchemy instead of plain SQL might not be obvious, especially considering the setup required to create the models representing the database. The results
returned by the query is where the magic happens. Instead of getting back a list of lists of scalar data, you’ll get back a list of instances of Author
objects with attributes matching the column names you defined.
The books
and publishers
collections maintained by SQLAlchemy create a hierarchical list of authors and the books they’ve written as well as the publishers who’ve published them.
Behind the scenes, SQLAlchemy turns the object and method calls into SQL statements to execute against the SQLite database engine. SQLAlchemy transforms the data returned by SQL queries into Python objects.
With SQLAlchemy, you can perform the more complex aggregation query shown earlier for the list of authors and the number of books they’ve written like this:
author_book_totals = (
session.query(
Author.first_name,
Author.last_name,
func.count(Book.title).label("book_total")
)
.join(Book)
.group_by(Author.last_name)
.order_by(desc("book_total"))
.all()
)
The query above gets the author’s first and last name, along with a count of the number of books that the author has written. The aggregating count
used by the group_by
clause is based on the author’s last name. Finally, the results are sorted in descending order based on the aggregated and aliased book_total
.
Example Program
The example program examples/example_2/main.py
has the same functionality as examples/example_1/main.py
but uses SQLAlchemy exclusively to interface with the author_book_publisher.db
SQLite database. The program is broken up into the main()
function and the functions it calls:
1def main():
2 """Main entry point of program"""
3 # Connect to the database using SQLAlchemy
4 with resources.path(
5 "project.data", "author_book_publisher.db"
6 ) as sqlite_filepath:
7 engine = create_engine(f"sqlite:///{sqlite_filepath}")
8 Session = sessionmaker()
9 Session.configure(bind=engine)
10 session = Session()
11
12 # Get the number of books printed by each publisher
13 books_by_publisher = get_books_by_publishers(session, ascending=False)
14 for row in books_by_publisher:
15 print(f"Publisher: {row.name}, total books: {row.total_books}")
16 print()
17
18 # Get the number of authors each publisher publishes
19 authors_by_publisher = get_authors_by_publishers(session)
20 for row in authors_by_publisher:
21 print(f"Publisher: {row.name}, total authors: {row.total_authors}")
22 print()
23
24 # Output hierarchical author data
25 authors = get_authors(session)
26 output_author_hierarchy(authors)
27
28 # Add a new book
29 add_new_book(
30 session,
31 author_name="Stephen King",
32 book_title="The Stand",
33 publisher_name="Random House",
34 )
35 # Output the updated hierarchical author data
36 authors = get_authors(session)
37 output_author_hierarchy(authors)
This program is a modified version of examples/example_1/main.py
. Let’s go over the differences:
-
Lines 4 to 7 first initialize the
sqlite_filepath
variable to the database file path. Then they create theengine
variable to communicate with SQLite and theauthor_book_publisher.db
database file, which is SQLAlchemy’s access point to the database. -
Line 8 creates the
Session
class from the SQLAlchemy’ssessionmaker()
. -
Line 9 binds the
Session
to the engine created in line 8. -
Line 10 creates the
session
instance, which is used by the program to communicate with SQLAlchemy.
The rest of the function is similar, except for the replacement of data
with session
as the first parameter to all the functions called by main()
.
get_books_by_publisher()
has been refactored to use SQLAlchemy and the models you defined earlier to get the data requested:
1def get_books_by_publishers(session, ascending=True):
2 """Get a list of publishers and the number of books they've published"""
3 if not isinstance(ascending, bool):
4 raise ValueError(f"Sorting value invalid: {ascending}")
5
6 direction = asc if ascending else desc
7
8 return (
9 session.query(
10 Publisher.name, func.count(Book.title).label("total_books")
11 )
12 .join(Publisher.books)
13 .group_by(Publisher.name)
14 .order_by(direction("total_books"))
15 )
Here’s what the new function, get_books_by_publishers()
, is doing:
-
Line 6 creates the
direction
variable and sets it equal to the SQLAlchemydesc
orasc
function depending on the value of theascending
Parameter. -
Lines 9 to 11 query the
Publisher
table for data to return, which in this case arePublisher.name
and the aggregate total ofBook
objects associated with an author, aliased tototal_books
. -
Line 12 joins to the
Publisher.books
Sammlung. -
Line 13 aggregates the book counts by the
Publisher.name
attribute. -
Line 14 sorts the output by the book counts according to the operator defined by
direction
. -
Line 15 closes the object, executes the query, and returns the results to the caller.
All the above code expresses what is wanted rather than how it’s to be retrieved. Now instead of using SQL to describe what’s wanted, you’re using Python objects and methods. What’s returned is a list of Python objects instead of a list of tuples of data.
get_authors_by_publisher()
has also been modified to work exclusively with SQLAlchemy. Its functionality is very similar to the previous function, so a function description is omitted:
def get_authors_by_publishers(session, ascending=True):
"""Get a list of publishers and the number of authors they've published"""
if not isinstance(ascending, bool):
raise ValueError(f"Sorting value invalid: {ascending}")
direction = asc if ascending else desc
return (
session.query(
Publisher.name,
func.count(Author.first_name).label("total_authors"),
)
.join(Publisher.authors)
.group_by(Publisher.name)
.order_by(direction("total_authors"))
)
get_authors()
has been added to get a list of authors sorted by their last names. The result of this query is a list of Author
objects containing a collection of books. The Author
objects already contain hierarchical data, so the results don’t have to be reformatted:
def get_authors(session):
"""Get a list of author objects sorted by last name"""
return session.query(Author).order_by(Author.last_name).all()
Like its previous version, add_new_book()
is relatively complex but straightforward to understand. It determines if a book with the same title, author, and publisher exists in the database already.
If the search query finds an exact match, then the function returns. If no book matches the exact search criteria, then it searches to see if the author has written a book using the passed in title. This code exists to prevent duplicate books from being created in the database.
If no matching book exists, and the author hasn’t written one with the same title, then a new book is created. The function then retrieves or creates an author and publisher. Once instances of the Book
, Author
and Publisher
exist, the relationships between them are created, and the resulting information is saved to the database:
1def add_new_book(session, author_name, book_title, publisher_name):
2 """Adds a new book to the system"""
3 # Get the author's first and last names
4 first_name, _, last_name = author_name.partition(" ")
5
6 # Check if book exists
7 book = (
8 session.query(Book)
9 .join(Author)
10 .filter(Book.title == book_title)
11 .filter(
12 and_(
13 Author.first_name == first_name, Author.last_name == last_name
14 )
15 )
16 .filter(Book.publishers.any(Publisher.name == publisher_name))
17 .one_or_none()
18 )
19 # Does the book by the author and publisher already exist?
20 if book is not None:
21 return
22
23 # Get the book by the author
24 book = (
25 session.query(Book)
26 .join(Author)
27 .filter(Book.title == book_title)
28 .filter(
29 and_(
30 Author.first_name == first_name, Author.last_name == last_name
31 )
32 )
33 .one_or_none()
34 )
35 # Create the new book if needed
36 if book is None:
37 book = Book(title=book_title)
38
39 # Get the author
40 author = (
41 session.query(Author)
42 .filter(
43 and_(
44 Author.first_name == first_name, Author.last_name == last_name
45 )
46 )
47 .one_or_none()
48 )
49 # Do we need to create the author?
50 if author is None:
51 author = Author(first_name=first_name, last_name=last_name)
52 session.add(author)
53
54 # Get the publisher
55 publisher = (
56 session.query(Publisher)
57 .filter(Publisher.name == publisher_name)
58 .one_or_none()
59 )
60 # Do we need to create the publisher?
61 if publisher is None:
62 publisher = Publisher(name=publisher_name)
63 session.add(publisher)
64
65 # Initialize the book relationships
66 book.author = author
67 book.publishers.append(publisher)
68 session.add(book)
69
70 # Commit to the database
71 session.commit()
The code above is relatively long. Let’s break the functionality down to manageable sections:
-
Lines 7 to 18 set the
book
variable to an instance of aBook
if a book with the same title, author, and publisher is found. Otherwise, they setbook
toNone
. -
Lines 20 and 21 determine if the book already exists and return if it does.
-
Lines 24 to 37 set the
book
variable to an instance of aBook
if a book with the same title and author is found. Otherwise, they create a newBook
Beispiel. -
Lines 40 to 52 set the
author
variable to an existing author, if found, or create a newAuthor
instance based on the passed-in author name. -
Lines 55 to 63 set the
publisher
variable to an existing publisher, if found, or create a newPublisher
instance based on the passed-in publisher name. -
Line 66 sets the
book.author
instance to theauthor
instance. This creates the relationship between the author and the book, which SQLAlchemy will create in the database when the session is committed. -
Line 67 adds the
publisher
instance to thebook.publishers
Sammlung. This creates the many-to-many relationship between thebook
andpublisher
tables. SQLAlchemy will create references in the tables as well as in thebook_publisher
association table that connects the two. -
Line 68 adds the
Book
instance to the session, making it part of the session’s unit of work. -
Line 71 commits all the creations and updates to the database.
There are a few things to take note of here. First, there’s is no mention of the author_publisher
or book_publisher
association tables in either the queries or the creations and updates. Because of the work you did in models.py
setting up the relationships, SQLAlchemy can handle connecting objects together and keeping those tables in sync during creations and updates.
Second, all the creations and updates happen within the context of the session
object. None of that activity is touching the database. Only when the session.commit()
statement executes does the session then go through its unit of work and commit that work to the database.
For example, if a new Book
instance is created (as in line 37 above), then the book has its attributes initialized except for the book_id
primary key and author_id
foreign key. Because no database activity has happened yet, the book_id
is unknown, and nothing was done in the instantiation of book
to give it an author_id
.
When session.commit()
is executed, one of the things it will do is insert book
into the database, at which point the database will create the book_id
primary key. The session will then initialize the book.book_id
value with the primary key value created by the database engine.
session.commit()
is also aware of the insertion of the Book
instance in the author.books
Sammlung. The author
object’s author_id
primary key will be added to the Book
instance appended to the author.books
collection as the author_id
foreign key.
Providing Access to Multiple Users
To this point, you’ve seen how to use pandas, SQLite, and SQLAlchemy to access the same data in different ways. For the relatively straightforward use case of the author, book, and publisher data, it could still be a toss-up whether you should use a database.
One deciding factor when choosing between using a flat file or a database is data and relationship complexity. If the data for each entity is complicated and contains many relationships between the entities, then creating and maintaining it in a flat file might become more difficult.
Another factor to consider is whether you want to share the data between multiple users. The solution to this problem might be as simple as using a sneakernet to physically move data between users. Moving data files around this way has the advantage of ease of use, but the data can quickly get out of sync when changes are made.
The problem of keeping the data consistent for all users becomes even more difficult if the users are remote and want to access the data across networks. Even when you’re limited to a single language like Python and using pandas to access the data, network file locking isn’t sufficient to ensure the data doesn’t get corrupted.
Providing the data through a server application and a user interface alleviates this problem. The server is the only application that needs file-level access to the database. By using a database, the server can take advantage of SQL to access the data using a consistent interface no matter what programming language the server uses.
The last example program demonstrates this by providing a web application and user interface to the Chinook sample SQLite database. Peter Stark generously maintains the Chinook database as part of the SQLite Tutorial site. If you’d like to learn more about SQLite and SQL in general, then the site is a great resource.
The Chinook database provides artist, music, and playlist information along the lines of a simplified Spotify. The database is part of the example code project in the project/data
folder.
Using Flask With Python, SQLite, and SQLAlchemy
The examples/example_3/chinook_server.py
program creates a Flask application that you can interact with using a browser. The application makes use of the following technologies:
-
Flask Blueprint is part of Flask and provides a good way to follow the separation of concerns design principle and create distinct modules to contain functionality.
-
Flask SQLAlchemy is an extension for Flask that adds support for SQLAlchemy in your web applications.
-
Flask_Bootstrap4 packages the Bootstrap front-end tool kit, integrating it with your Flask web applications.
-
Flask_WTF extends Flask with WTForms, giving your web applications a useful way to generate and validate web forms.
-
python_dotenv is a Python module that an application uses to read environment variables from a file and keep sensitive information out of program code.
Though not necessary for this example, a .env
file holds the environment variables for the application. The .env
file exists to contain sensitive information like passwords, which you should keep out of your code files. However, the content of the project .env
file is shown below since it doesn’t contain any sensitive data:
SECRET_KEY = "you-will-never-guess"
SQLALCHEMY_TRACK_MODIFICATIONS = False
SQLAlCHEMY_ECHO = False
DEBUG = True
The example application is fairly large, and only some of it is relevant to this tutorial. For this reason, examining and learning from the code is left as an exercise for the reader. That said, you can take a look at an animated screen capture of the application below, followed by the HTML that renders the home page and the Python Flask route that provides the dynamic data.
Here’s the application in action, navigating through various menus and features:
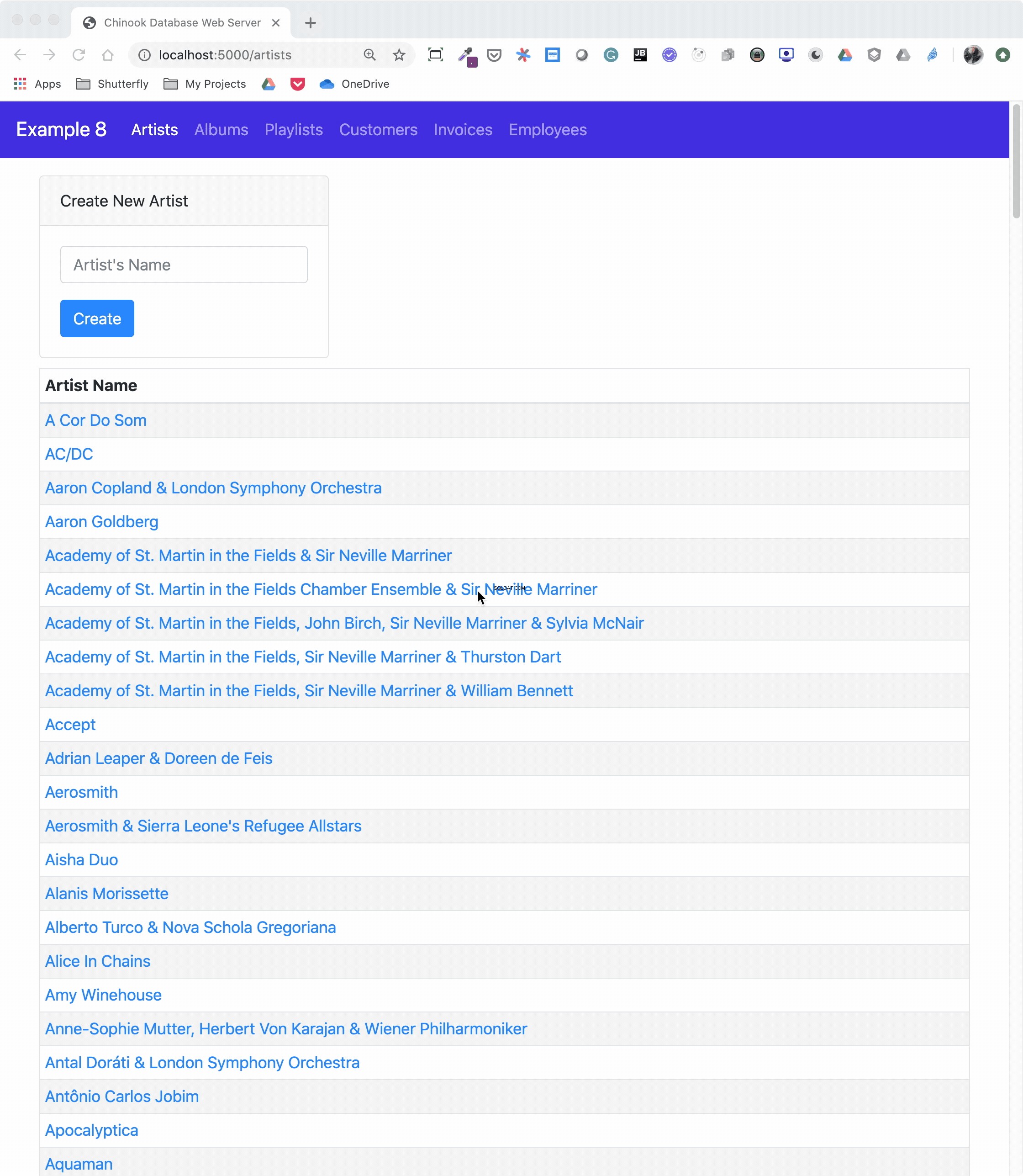
The animated screen capture starts on the application home page, styled using Bootstrap 4. The page displays the artists in the database, sorted in ascending order. The remainder of the screen capture presents the results of clicking on the displayed links or navigating around the application from the top-level menu.
Here’s the Jinja2 HTML template that generates the home page of the application:
1{% extends "base.html" %}
2
3{% block content %}
4<div class="container-fluid">
5 <div class="m-4">
6 <div class="card" style="width: 18rem;">
7 <div class="card-header">Create New Artist</div>
8 <div class="card-body">
9 <form method="POST" action="{{url_for('artists_bp.artists')}}">
10 {{ form.csrf_token }}
11 {{ render_field(form.name, placeholder=form.name.label.text) }}
12 <button type="submit" class="btn btn-primary">Create</button>
13 </form>
14 </div>
15 </div>
16 <table class="table table-striped table-bordered table-hover table-sm">
17 <caption>List of Artists</caption>
18 <thead>
19 <tr>
20 <th>Artist Name</th>
21 </tr>
22 </thead>
23 <tbody>
24 {% for artist in artists %}
25 <tr>
26 <td>
27 <a href="{{url_for('albums_bp.albums', artist_id=artist.artist_id)}}">
28 {{ artist.name }}
29 </a>
30 </td>
31 </tr>
32 {% endfor %}
33 </tbody>
34 </table>
35 </div>
36</div>
37{% endblock %}
Here’s what’s going on in this Jinja2 template code:
-
Line 1 uses Jinja2 template inheritance to build this template from the
base.html
template. Thebase.html
template contains all the HTML5 boilerplate code as well as the Bootstrap navigation bar consistent across all pages of the site. -
Lines 3 to 37 contain the block content of the page, which is incorporated into the Jinja2 macro of the same name in the
base.html
base template. -
Lines 9 to 13 render the form to create a new artist. This uses the features of Flask-WTF to generate the form.
-
Lines 24 to 32 create a
for
loop that renders the table of artist names. -
Lines 27 to 29 render the artist name as a link to the artist’s album page showing the songs associated with a particular artist.
Here’s the Python route that renders the page:
1from flask import Blueprint, render_template, redirect, url_for
2from flask_wtf import FlaskForm
3from wtforms import StringField
4from wtforms.validators import InputRequired, ValidationError
5from app import db
6from app.models import Artist
7
8# Set up the blueprint
9artists_bp = Blueprint(
10 "artists_bp", __name__, template_folder="templates", static_folder="static"
11)
12
13def does_artist_exist(form, field):
14 artist = (
15 db.session.query(Artist)
16 .filter(Artist.name == field.data)
17 .one_or_none()
18 )
19 if artist is not None:
20 raise ValidationError("Artist already exists", field.data)
21
22class CreateArtistForm(FlaskForm):
23 name = StringField(
24 label="Artist's Name", validators=[InputRequired(), does_artist_exist]
25 )
26
27@artists_bp.route("/")
28@artists_bp.route("/artists", methods=["GET", "POST"])
29def artists():
30 form = CreateArtistForm()
31
32 # Is the form valid?
33 if form.validate_on_submit():
34 # Create new artist
35 artist = Artist(name=form.name.data)
36 db.session.add(artist)
37 db.session.commit()
38 return redirect(url_for("artists_bp.artists"))
39
40 artists = db.session.query(Artist).order_by(Artist.name).all()
41 return render_template("artists.html", artists=artists, form=form,)
Let’s go over what the above code is doing:
-
Lines 1 to 6 import all the modules necessary to render the page and initialize forms with data from the database.
-
Lines 9 to 11 create the blueprint for the artists page.
-
Lines 13 to 20 create a custom validator function for the Flask-WTF forms to make sure a request to create a new artist doesn’t conflict with an already existing artist.
-
Lines 22 to 25 create the form class to handle the artist form rendered in the browser and provide validation of the form field inputs.
-
Lines 27 to 28 connect two routes to the
artists()
function they decorate. -
Line 30 creates an instance of the
CreateArtistForm()
class. -
Line 33 determines if the page was requested through the HTTP methods GET or POST (submit). If it was a POST, then it also validates the fields of the form and informs the user if the fields are invalid.
-
Lines 35 to 37 create a new artist object, add it to the SQLAlchemy session, and commit the artist object to the database, persisting it.
-
Line 38 redirects back to the artists page, which will be rerendered with the newly created artist.
-
Line 40 runs an SQLAlchemy query to get all the artists in the database and sort them by
Artist.name
. -
Line 41 renders the artists page if the HTTP request method was a GET.
You can see that a great deal of functionality is created by a reasonably small amount of code.
Creating a REST API Server
You can also create a web server providing a REST API. This kind of server offers URL endpoints responding with data, often in JSON format. A server providing REST API endpoints can be used by JavaScript single-page web applications through the use of AJAX HTTP requests.
Flask is an excellent tool for creating REST applications. For a multi-part series of tutorials about using Flask, Connexion, and SQLAlchemy to create REST applications, check out Python REST APIs With Flask, Connexion, and SQLAlchemy.
If you’re a fan of Django and are interested in creating REST APIs, then check out Django Rest Framework – An Introduction and Create a Super Basic REST API with Django Tastypie.
Hinweis: It’s reasonable to ask if SQLite is the right choice as the database backend to a web application. The SQLite website states that SQLite is a good choice for sites that serve around 100,000 hits per day. If your site gets more daily hits, the first thing to say is congratulations!
Beyond that, if you’ve implemented your website with SQLAlchemy, then it’s possible to move the data from SQLite to another database such as MySQL or PostgreSQL. For a comparison of SQLite, MySQL, and PostgreSQL that will help you make decisions about which one will serve your application best, check out Introduction to Python SQL Libraries.
It’s well worth considering SQLite for your Python application, no matter what it is. Using a database gives your application versatility, and it might create surprising opportunities to add additional features.
Conclusion
You’ve covered a lot of ground in this tutorial about databases, SQLite, SQL, and SQLAlchemy! You’ve used these tools to move data contained in flat files to an SQLite database, access the data with SQL and SQLAlchemy, and provide that data through a web server.
In this tutorial, you’ve learned:
- Why an SQLite database can be a compelling alternative to flat-file data storage
- How to normalize data to reduce data redundancy and increase data integrity
- How to use SQLAlchemy to work with databases in an object-oriented manner
- How to build a web application to serve a database to multiple users
Working with databases is a powerful abstraction for working with data that adds significant functionality to your Python programs and allows you to ask interesting questions of your data.
You can get all of the code and data you saw in this tutorial at the link below:
Download the sample code: Click here to get the code you’ll use to learn about data management with SQLite and SQLAlchemy in this tutorial.
Further Reading
This tutorial is an introduction to using databases, SQL, and SQLAlchemy, but there’s much more to learn about these subjects. These are powerful, sophisticated tools that no single tutorial can cover adequately. Here are some resources for additional information to expand your skills:
-
If your application will expose the database to users, then avoiding SQL injection attacks is an important skill. For more information, check out Preventing SQL Injection Attacks With Python.
-
Providing web access to a database is common in web-based single-page applications. To learn how, check out Python REST APIs With Flask, Connexion, and SQLAlchemy – Part 2.
-
Preparing for data engineering job interviews gives you a leg up in your career. To get started, check out Data Engineer Interview Questions With Python.
-
Migrating data and being able to roll back using Flask with Postgres and SQLAlchemy is an integral part of the Software Development Life Cycle (SDLC). You can learn more about it by checking out Flask by Example – Setting up Postgres, SQLAlchemy, and Alembic.